Anúncios
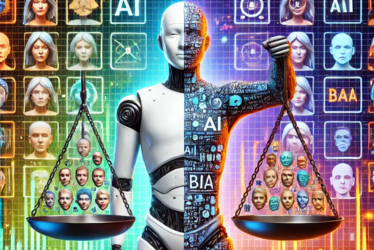
Are We Predicting or Prejudging?
Imagine this scenario: an artificial intelligence system is used to predict the next economic crisis or identify the communities most affected by a natural disaster.
Sounds fantastic, right? But what if these systems end up making decisions based on historical biases or reinforcing stereotypes? What if, instead of helping, the technology is exacerbating inequalities?
This is the core discussion surrounding algorithmic bias, a growing concern as AI becomes indispensable in various fields. While the idea that technology is impartial is widely promoted, the reality is that algorithms merely reproduce what they learn—and they often learn from data filled with biases.
In this article, we will explore how artificial intelligence can perpetuate inequalities by analyzing real cases, reviewing expert studies, and investigating solutions to ensure AI serves as a force for good rather than another cog in the wheel of social injustices.
The Paradox of Neutrality in Artificial Intelligence
et’s get straight to the point: artificial intelligence is not neutral. Designers build systems to process information objectively, but these systems directly reflect the data used to train them.
A Bloomberg analysis highlights that algorithms inevitably carry the marks of historical biases. Engineers don’t deliberately create bias; instead, the inequalities come from the real-world data itself.
Take, for instance, a system designed to predict the impacts of an earthquake. This system must decide where to allocate resources. It will analyze historical data, but if that data shows that affluent neighborhoods received more aid in the past, the algorithm might simply “learn” that these areas are higher priorities—completely overlooking vulnerable communities that were previously neglected.
What’s fascinating here is how technology both solves problems and creates them. When built on a solid data foundation and clear objectives, AI predicts crises effectively. Otherwise, as practice shows, AI simply perpetuates inequalities.
Want to learn more about how AI can perpetuate inequalities? Read the full article to discover the consequences and possible solutions!
Stereotypes Embedded in Algorithms
One of the most concerning aspects of algorithmic bias is how it can amplify cultural and racial stereotypes, sometimes in ways that are nearly invisible. For example, Wired highlighted how generative AI systems, such as chatbots or language models, tend to reproduce gender and racial biases.
This happens because these tools learn from vast datasets, many of which are riddled with examples of discrimination.
A well-known case involves the use of AI in predictive policing. Here, the algorithm analyzes crime patterns and attempts to anticipate where new crimes might occur.
The problem? These systems often recommend increased patrols in predominantly Black or low-income neighborhoods. This is no coincidence—it’s a reflection of historical policing data, which was already biased.
And there’s more. A study discussed by Forbes revealed that AI, when predicting crises or behaviors, often attributes negative or “risky” behaviors to certain racial or socioeconomic groups. This not only creates stigma but also shapes public policies that reinforce cycles of exclusion.
At the end of the day, AI isn’t creating these stereotypes from scratch—it’s simply reproducing what humans have done in the past. But for many, this makes the issue even more troubling.
After all, an algorithm may appear impartial, but its decisions can directly and unequally impact lives.
How Algorithmic Bias Affects Crisis Prediction
Let’s be honest: predicting crises is no easy task. To do so, artificial intelligence systems must work with historical data, much of which was neither collected nor analyzed with social justice in mind. The result? Biased predictions.
Chapman University highlights this issue clearly: if an algorithm is trained on data that neglects minorities or marginalized communities, it will inevitably replicate those shortcomings.
Take, for instance, a system designed to predict which regions will be most affected by a public health crisis. If historical data shows that wealthy communities received more investment in health infrastructure, the algorithm might overlook the urgent needs of underserved areas.
The problem here isn’t just technical; it’s ethical. Are we truly comfortable with the idea that a history of inequality should dictate the future?
Learn more about the ethical challenges of AI in crisis prediction.
Adopting AI in Businesses: A Matter of Priorities and Risks
Artificial intelligence is being widely adopted across sectors such as telecommunications, financial services, and automotive industries, but this expansion doesn’t always come with a critical view of the risks involved.
The chart below, based on a McKinsey survey, shows that while most companies acknowledge concerns like cybersecurity, ethical issues such as privacy and fairness still go largely unnoticed by the majority.
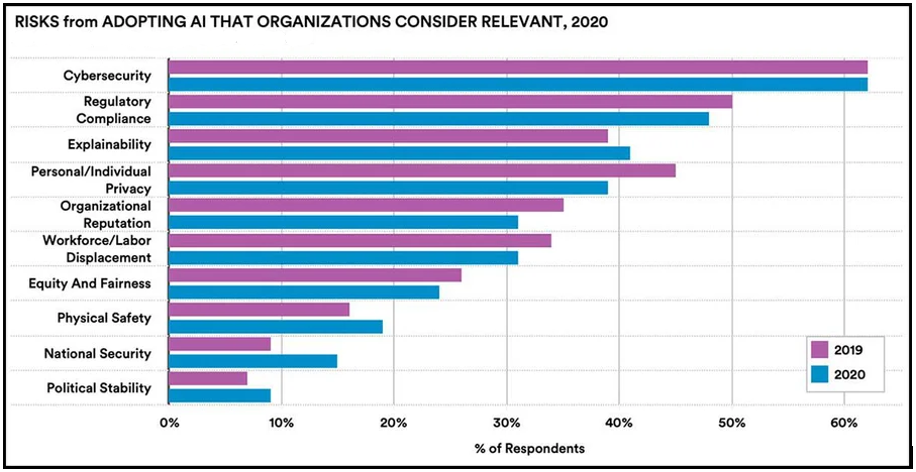
This data highlights a significant gap between academic research, which has been actively debating topics like algorithmic bias and AI fairness, and business practices.
While researchers place these issues at the forefront of discussions, many corporations seem to lack a full understanding of AI’s ethical impacts on their operations.
What does this mean? Despite the rapid adoption of AI, there is still a lack of urgency in integrating ethical considerations and ensuring that these technologies are used fairly and responsibly.
Baseball and Other Stories of Algorithmic Bias
Looking for a more unexpected yet equally revealing example? Consider the use of artificial intelligence in sports, particularly baseball. According to an article in The Guardian, automated scouting systems in Major League Baseball (MLB) often prioritize metrics that favor players from higher socioeconomic backgrounds, who are frequently white.
This has led to the exclusion of talented Black and Latino players, many of whom come from less privileged backgrounds with limited access to advanced sports resources.
As distant as this might seem, it’s a crucial example for understanding how algorithmic bias works. The algorithm analyzes the data it’s given and makes decisions based on it.The problem is that the data is far from neutral.
Sports mirror what happens in other areas, such as corporate talent recruitment, financial credit approval, and public policy. The common thread? AI predicts “potential” or “risk” but often excludes those who need opportunities the most.
Explore how AI-driven metrics in baseball highlight systemic biases, impacting Black and Latino players in the MLB:
Generative AI and Visual Biases
Beyond predictions, another area gaining attention is image generation through AI. Tools like DALL-E and MidJourney are revolutionizing how we create art and visualize the world. However, as highlighted by Science News Explorers, these systems also carry biases.
For instance, when asked to generate images of professionals, many algorithms associate doctors with white men and nurses with women.
Similarly, requests for representations of “leadership” predominantly produce male figures. These seemingly minor details are anything but insignificant—they shape how we perceive the world, reinforcing visual stereotypes that influence everything from trust in professions to media portrayals.
This issue becomes particularly concerning in the context of crises. AI-generated images or visual representations steeped in bias directly impact how people and organizations respond to events, perpetuating inequalities in critical moments.
How to Correct the Course? Solutions to Algorithmic Bias
Despite the challenges, there are ways to combat algorithmic bias and turn artificial intelligence into an ally for a fairer future. Here are some ideas based on the studies we’ve analyzed:
- Continuous Audits: As highlighted by Wired, regularly reviewing algorithms for biases is essential. This involves not only identifying obvious errors but also questioning the assumptions embedded in the data.
- Diverse Teams: Chapman University emphasizes that diverse teams—in terms of race, gender, and experience—are crucial for spotting biases that might go unnoticed in homogeneous groups.
- Hybrid Systems: InData Labs suggests that including humans in the review process is vital, especially for decisions involving ethical or cultural considerations.
- Education and Awareness: Part of the issue is that many developers and organizations still don’t fully understand the impact of algorithmic bias. Awareness campaigns can help bridge this gap.
- Transparency: Companies developing AI must be more transparent about how their systems operate, what data they use, and what efforts are in place to mitigate bias.
These solutions are not only practical but also a step toward ensuring that AI serves as a force for equity rather than a perpetuator of inequalities.
Organizations and the Challenge of Bias in AI
A revealing insight into how companies are addressing algorithmic bias comes from an analysis by Datanami, highlighting a concerning landscape.
The chart below illustrates the primary challenges organizations face in mitigating bias in artificial intelligence systems.
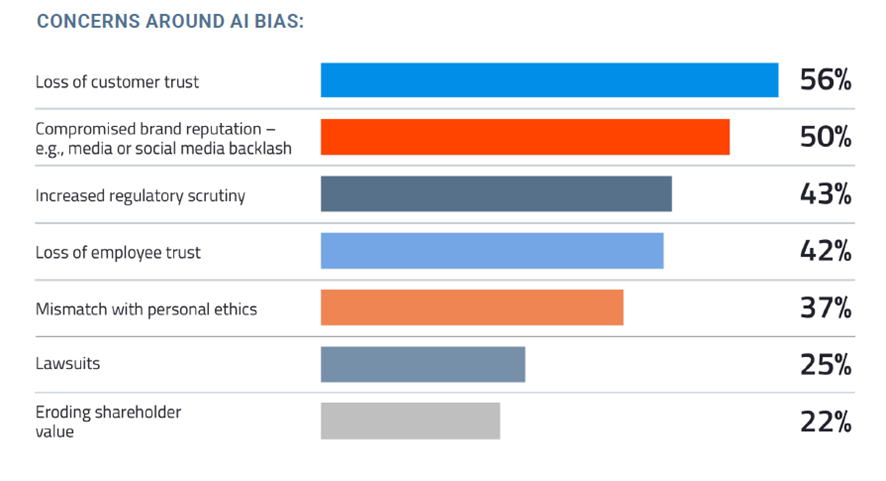
The data shows that, while many companies acknowledge the issue, few have effective processes in place to address it. Key barriers include lack of transparency, insufficient training, and homogeneous teams.
What does this tell us? That algorithmic bias is not merely a technical problem but also an organizational and strategic one. To ensure AI serves as an ally rather than a magnifier of inequalities, companies must invest in diversity, training, and continuous audits.
The Growth of the Ethical Debate in Artificial Intelligence
Although many corporations are still lagging behind when it comes to ethics in AI, researchers have made significant strides in this area. The chart below highlights the sharp increase in academic papers related to AI ethics presented at conferences in recent years.
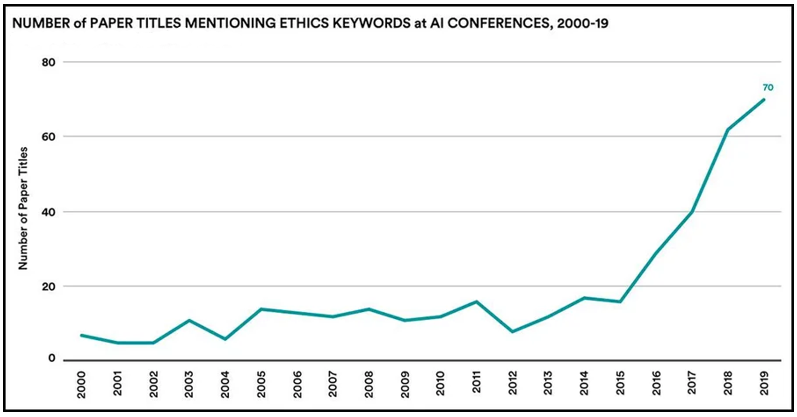
This is a positive sign that issues like algorithmic bias, opacity in decision-making, and privacy are gaining more attention among experts.
As noted by AI Index, this growing interest at conferences indicates that future generations of professionals will be more focused on addressing these ethical challenges.
The report emphasizes that researchers are only beginning to develop quantitative bias testing in AI systems. In other words, while progress is underway, much work remains to translate academic awareness into practical, scalable solutions.
Can We Create a Fairer Artificial Intelligence?
In the end, the debate about artificial intelligence and algorithmic bias is not just technical – it’s deeply human.
We are talking about how we use technology to shape the future and how we ensure that it is inclusive and fair for everyone.
The good news is that we have the tools and knowledge to fix these issues. The bad news? It requires effort, commitment, and a shift in mindset about what we consider “normal” in technology.
If we want to use artificial intelligence to predict crises ethically and effectively, we must ensure that it doesn’t simply repeat the mistakes of the past. After all, the future should be a place where innovation doesn’t mean exclusion – and where everyone has a chance to thrive.