Anúncios
Have you ever imagined a system that can “learn” on its own from an enormous amount of data and improve as it receives more information?
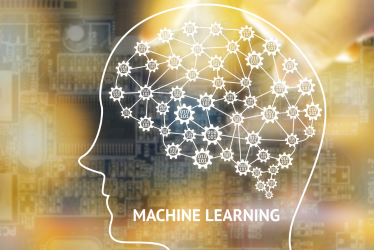
That’s Machine Learning.
This branch of artificial intelligence enables computers to analyze patterns and detect anomalies without relying on fixed instructions. Instead of depending on predefined rules, ML algorithms adapt and become increasingly effective.
Think of a tool that monitors financial transactions around the world. With Machine Learning, it can identify subtle signals, such as changes in purchasing behavior or fluctuations in interest rates, which could go unnoticed in traditional analyses. These signals, when connected, might be the necessary warning to anticipate an impending crisis.
Traditional Methods: Limitations and Challenges
Before the revolution of artificial intelligence, economists primarily relied on traditional methods, such as statistical analyses and equation-based models. While these methods served well for many years, they face significant challenges in an increasingly complex and digitized world:
- Dependence on Historical Data: Traditional models base their predictions on the past, which is problematic since crises are often triggered by unexpected and novel factors.
- Difficulty with Big Data: With today’s massive volume of available data, older approaches simply lack the capacity to process and interpret such varied and voluminous information.
- Inability to Capture Human Behavior: Financial crises are often driven by human emotions, like panic and euphoria, which are extremely difficult to measure using traditional statistical models.
Comparison: Machine Learning vs. Traditional Methods
While ML is surpassing traditional methods in many aspects, it’s helpful to understand their differences in a practical context.
Characteristic | Machine Learning | Traditional Methods |
Speed | Real-time processing | Reliant on manual analysis |
Adaptability | Highly adjustable to new data | Rigid initial assumptions |
Data Coverage | Covers Big Data and diverse sources | Limited to structured data |
Human Focus | Integrates human and psychological behavior | Based solely on economic histories |
How Machine Learning Transforms Crisis Prediction
If traditional methods are like old maps, Machine Learning (ML) is a modern GPS that adjusts in real-time. Here are some ways ML is revolutionizing financial crisis prediction:
- Real-Time Analysis: While traditional methods may take months to produce insights, ML algorithms analyze data in real time, enabling quicker responses to warning signs.
- Identification of Complex Patterns: ML uncovers connections invisible to conventional analyses, such as the relationship between climate change and impacts on the agricultural sector, which may influence broader economic crises.
- Anticipation of Human Behavior: Models trained on data from social media and internet searches can predict large-scale human reactions. For example, a significant increase in negative comments about economic conditions could be a clear warning sign of an approaching crisis.
Real-World Examples: Machine Learning in Practice
The use of Machine Learning is bringing a fresh perspective to industries once limited by traditional methods. Here are some real examples of how this technology is being used to predict financial crises and manage risks:
The Case of the Real Estate Market
After the 2008 financial crisis, which originated in the mortgage market, Machine Learning tools became widely used to monitor real estate lending data. For example:
- Black Knight Financial Services, a major U.S. analytics company, developed ML-powered tools to analyze mortgage delinquency rates and detect early signs of instability.
- These systems help banks and investors identify at-risk markets before new housing bubbles form, allowing for more proactive risk management.
Visit Black Knight’s page on data analytics for the housing market:
Stock Market Volatility Prediction
Modern financial platforms rely on ML algorithms to forecast stock market fluctuations. They process variables such as trading volumes, social media sentiment, and trends in global economic news. This method equips investors with sharper insights into market behavior.
Risk Management in Banking
Major financial institutions like JPMorgan and Goldman Sachs have implemented ML in their risk operations. These algorithms help predict defaults, identify systemic risks, and quickly adjust asset portfolios, minimizing potential losses.
Benefits of Machine Learning in Crisis Prediction
The application of Machine Learning in economics goes far beyond isolated examples. It offers significant benefits that surpass the limitations of traditional methods:
- Increased Accuracy:
By minimizing human error, ML algorithms provide more reliable predictions based on detailed analyses. - Speed:
ML processes large volumes of data in seconds, enabling governments and businesses to respond quickly to changes and warning signs. - Flexibility:
ML models are dynamic and can be adjusted in real-time to include new variables or adapt to emerging scenarios.
Challenges of Machine Learning in Crisis Prediction
While Machine Learning (ML) offers a revolutionary approach, it is not without flaws and faces significant challenges that must be addressed to reach its full potential:
- Dependence on High-Quality Data:
An algorithm is only as good as the data it’s trained on. Inaccurate, incomplete, or biased information can lead to incorrect predictions, putting critical decisions at risk. - Interpretation of Results:
Although ML identifies complex patterns, its algorithms often fail to explain the “why” behind their findings. This lack of transparency can make it difficult to interpret results and make strategic decisions. - Ethical Risks:
The use of sensitive data and privacy concerns raise ethical questions. Companies and governments must ensure that ML applications respect privacy and are used fairly and responsibly.
The Future of Machine Learning in Economics
The future of Machine Learning in financial crisis prediction promises to be even more impactful, with innovations that will enhance its efficiency and accessibility:
- Integration with Blockchain:
The combination of ML with blockchain can create more transparent and secure economic models. This integration enables greater traceability and reliability in the data being analyzed. - Explainable AI:
“Explainable AI” technologies are being developed to improve the interpretability of ML algorithms. This will help economists and decision-makers better understand results, increasing trust in the technology. - Collaboration Between Humans and Machines:
Rather than replacing economists, ML is evolving as a collaborative tool. It combines large-scale data analysis with human judgment, enabling more accurate predictions and better-informed decisions.
How Machine Learning Detects Early Signs of Crises
The key differentiator of Machine Learning (ML) lies in its ability to detect signs of crises before they become apparent. This technology uses historical and real-time data to identify patterns that could precede financial crises.
Big Data Processing: ML algorithms can analyze billions of financial transactions, detect anomalies, and correlate them with historical crisis events. This provides insights that traditional models simply cannot achieve.
Machine Learning and Sentiment Analysis in Economics
The integration of ML with sentiment analysis has driven significant advancements in financial crisis prediction. This combination allows for monitoring not only economic data but also the “mood” of the markets.
How It Works:
Social Media: ML analyzes sentiment expressed on platforms like Twitter and LinkedIn to capture signals of optimism or pessimism in the markets.
News and Publications: Tools like Natural Language Processing (NLP) evaluate the emotional impact of economic headlines, helping predict how investors might react.
Example: Sentiment analysis was widely used during the COVID-19 pandemic to interpret social and economic data. AI-based tools analyzed the surge in terms like “recession” and “market crash” on Twitter, news publications, and economic reports, helping to forecast economic trends.
Emerging Applications of Machine Learning in Economic Forecasting
The use of ML goes far beyond predicting financial crises. It is being applied across a wide range of economic contexts:
Corporate Sustainability Monitoring:
Companies are using ML to predict how economic crises might impact sustainability practices, enabling strategic adjustments.
Government Fiscal Planning:
Governments have implemented ML-based models to forecast tax revenues and adjust budgetary policies, minimizing the effects of economic crises.
Example:
During the COVID-19 pandemic, governments, including India, leveraged Machine Learning algorithms to forecast tax revenues and adjust fiscal policies. By analyzing economic data and trends in real-time, these tools helped allocate subsidies to vulnerable populations, mitigating the pandemic’s economic impact.
The Relationship Between Machine Learning and Economic Sustainability
achine Learning is playing a critical role in fostering economic sustainability by addressing climate challenges. For instance, ML models are being used to predict climate-related risks, optimize resource allocation, and propose solutions that strengthen economic resilience while supporting sustainable development goals.
Example:United Nations Framework Convention on Climate Change (UNFCCC) The report outlines how Machine Learning applications help analyze environmental data to guide sustainable economic decisions, reduce emissions, and support resilient infrastructure development.
Machine Learning and the New Era of Economics
Machine Learning is not just a technological evolution; it is a paradigm shift in how we understand and tackle financial crises. By surpassing the limitations of traditional methods, it offers more accurate predictions, faster analyses, and an unprecedented ability to adapt. However, its success hinges on crucial elements: reliable data, regulations that ensure transparency, and ethical usage that respects privacy and individual rights.
As we face an economic future filled with uncertainty, ML emerges as more than just a forecasting tool—it is a strategic ally to navigate challenging times with confidence and resilience. After all, understanding what lies ahead is not just a competitive advantage but a necessity to thrive in a constantly changing world.
References
GOODFELLOW, Ian; BENGIO, Yoshua; COURVILLE, Aaron. Deep Learning. Cambridge: MIT Press, 2016.
IBM. What is Machine Learning?
UNFCCC. Artificial Intelligence for Climate Action: Applications and Opportunities. 2020.
SPRINGERLINK. Machine learning and economic forecasting: Techniques and implications. 2021.