Anúncios
Did you know that the emotions and opinions shared daily, whether on social media or in the news, can impact the economy?
Teste teste teste teste
teste de bloco
That’s where Sentiment Analysis comes in—a groundbreaking technique that combines technology and human behavior to capture the “mood” of markets and predict economic trends. This tool not only transforms economic reports but also helps us understand the future in ways that once seemed impossible.
But what exactly is this sentiment analysis? And how can it shape the future of the economy? Let’s break it down together.
What is Sentiment Analysis?
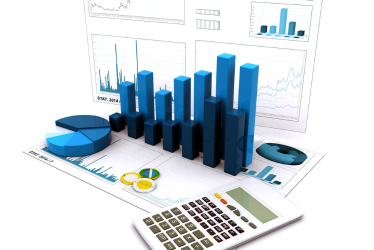
Have you ever thought about being able to “sense” the mood of a market or how people feel about a particular topic? That’s precisely what sentiment analysis does. Using artificial intelligence and algorithms, it interprets the emotional tone present in texts such as news articles, social media posts, and even speeches.
Simply put, it works like “emotion reading,” categorizing texts into three main groups:
- Positive: When there’s optimism or enthusiasm.
- Negative: When signs of pessimism or concern appear.
- Neutral: When the tone is more balanced or factual.
In the world of economics, it goes beyond interpreting emotions—it’s about understanding how investors, consumers, and businesses feel about specific topics. These sentiments, as we’ll see ahead, are crucial pieces in creating predictive analyses that help forecast future scenarios.
How is Sentiment Analysis Transforming Economic Reports?
Traditional economic reports have always relied on numbers and historical data. They were important, of course, but something was missing: human behavior. Now, with sentiment analysis, these reports have evolved into something far more dynamic and comprehensive, capable of capturing the emotions and trends behind the data. Here’s how this happens in practice:
1. Anticipating Movements in the Financial Market
Imagine a single tweet completely changing the fate of an asset. Remember the famous “Elon Musk effect”? A single comment from him has been enough to send cryptocurrencies skyrocketing or plummeting. Sentiment analysis helps identify, in real time, the impact of such statements on the market.
Real Example:
During the pandemic, investors turned to social media platforms like Twitter and Reddit to discuss stocks. Sentiment analysis tools captured the enthusiasm around GameStop, helping predict the surprising surge in the company’s stock value.
2. More Accurate Predictions with Predictive Analysis
When you combine sentiment analysis with historical data, you get something powerful: predictive analysis. This allows for more accurate forecasts of market fluctuations, changes in consumer behavior, and even economic crises.
Practical Example:
During periods of rising prices, social media data analysis can reveal crucial patterns regarding how consumers are perceiving the increase in the cost of living. Recently, specialized companies monitored a surge in negative comments on social media, signaling discontent with inflation. By cross-referencing this data with traditional economic indicators, it was possible to predict an increase in inflation in specific sectors.
A fascinating study titled “Sentiment Analysis on Inflation after COVID-19“ explored how sentiment analysis of tweets can anticipate inflation changes. Using machine learning models, the researchers analyzed social media discussions about inflation, identifying patterns that indicate future inflationary trends.
Additionally, the article “Using Twitter Data to Measure Inflation Perception“ demonstrates how public perceptions, captured through social media sentiment, can complement traditional economic data. This provides a more dynamic and detailed view of inflation expectations, enabling more assertive decision-making.
These examples showcase the immense potential of sentiment analysis, particularly when combined with economic data, to provide valuable insights into inflationary trends in specific sectors.
3. Monitoring Economic Risks
Financial crises are often preceded by waves of negative discussions, whether about political instability, sectoral problems, or economic uncertainties. Sentiment analysis monitors these conversations and can alert economists to act before a crisis unfolds.
Case Study:
During the 2007–2008 global financial crisis, early warning signals were identified through various indicators, including market sentiment. A study titled “Who Knew: Financial Crises and Investor Sentiment” analyzed the World Equity Market Sentiment Index (WEMSI) and found that while market sentiment was high in early 2008, it began to decline and turn negative several months before the collapse of Lehman Brothers.
Additionally, research published in the journal Scientific Reports examined early signs of the crisis in world trade data. The study highlighted that while some early-warning signals were present, they were not sufficient to predict the crisis accurately.
These findings suggest that incorporating sentiment analysis into early warning systems could have potentially identified the impending crisis sooner, allowing for more timely interventions.
How Does Sentiment Analysis Work in Practice?
Sentiment analysis acts as a digital filter capable of interpreting the emotional tone present in texts. This technology uses sophisticated Natural Language Processing (NLP) algorithms to understand the context and meaning of words, transforming them into structured and organized data for analysis.
1. Data Collection
It all starts with gathering information. Algorithms scour various sources in search of relevant data, such as:
- Economic and financial news.
- Social media posts, where the public expresses opinions in real-time.
- Corporate reports, which often hide valuable market clues.
2. Classification
Once collected, the data is categorized into three main groups:
- Positive: Reflects optimism or confidence.
- Negative: Indicates concern or warning.
- Neutral: Presents a factual and balanced tone.
It’s as if the algorithms separate enthusiasm from pessimism, allowing for a clear understanding of the overall mood.
3. Analysis
In the final stage, the categorized data is analyzed to identify patterns and trends. These insights help predict the impact of events, corporate decisions, or even future economic crises.
Challenges Faced by Sentiment Analysis
Despite being an extremely useful technology, sentiment analysis still deals with various limitations and challenges that must be overcome to achieve its full potential.
Cultural and Linguistic Context
Words can take on different meanings depending on the context in which they’re used or the cultural differences associated with them. A term might be optimistic in one place but negative in another. This challenge can lead to misinterpretations if algorithms aren’t adjusted to capture regional nuances.
Sarcasm and Irony
Imagine someone writing: “Oh, sure, this economic plan is going to save the world!” Sarcasm often goes unnoticed by many algorithms, which can distort the results by interpreting the statement as positive.
The Future Role of Sentiment Analysis in Economic Reporting
With the continuous evolution of artificial intelligence, the potential of sentiment analysis is only just beginning to be explored. What was once accessible only to large financial institutions is now expanding to small businesses and even local governments. In the future, it will become an indispensable tool, aiding everything from strategic decisions to detailed economic forecasts.
Imagine a scenario where a small business can predict changes in consumer behavior in real-time or a government uses sentiment analysis to anticipate social and economic crises. This is the path we are heading down—a more informed and adaptable economy.
The Relevance of Sentiment Analysis in the Global Economy
In an increasingly connected world, sentiment analysis is not just an optional tool—it has become a strategic necessity. In 2023, a McKinsey study revealed that more than 70% of leading financial firms are investing in sentiment analysis technologies to gain a competitive edge. But why is this so important?
Connection to New Economic Dynamics
The modern economy is heavily influenced by intangible variables such as consumer confidence, political volatility, and market fluctuations. Sentiment analysis helps decode these influences, allowing businesses and governments to act quickly.
Application in Public Policy
Beyond its application in financial markets, governments are using sentiment analysis to gauge public perception of economic policies, such as tax increases or subsidies. This enables them to adjust their approaches before a public relations crisis undermines their initiatives.
Sentiment Analysis Applied to Specific Economic Sectors
The application of sentiment analysis varies by sector, but the outcomes are equally transformative:
In the Financial Sector
Banks and brokerages use sentiment analysis to identify patterns that may predict market changes. For example, an increase in negative comments about a stock could signal an impending sell-off.
In Retail and E-Commerce
Companies like Amazon and Alibaba analyze consumer sentiment to adjust prices, identify popular products, and enhance the customer experience.
In the Entertainment Industry
Streaming platforms monitor feedback to fine-tune their content catalogs. For instance, Netflix uses sentiment analysis to predict the success of future productions.
The adoption of sentiment analysis differs significantly across economic sectors. The following chart illustrates the percentage of companies in each sector that have implemented this technology in their operations:
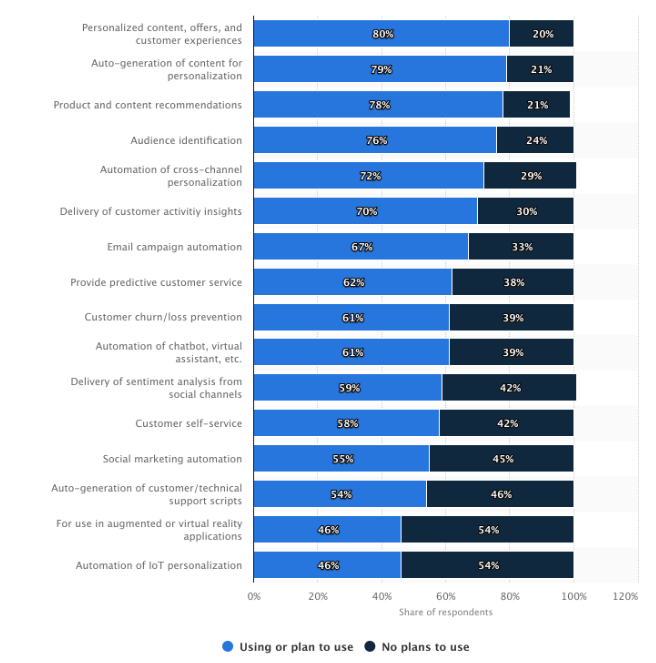
As we can see, sectors such as technology and financial services lead in the implementation of sentiment analysis, reflecting their pursuit of deeper insights into customer behavior. On the other hand, traditional industries such as manufacturing and construction show more modest adoption, indicating significant opportunities for applying this technology and achieving competitive advantages.
Technologies That Enhance Sentiment Analysis
The effectiveness of sentiment analysis is amplified when combined with other advanced technologies, creating more precise and comprehensive solutions.
Big Data
The massive volume of data generated daily provides a solid foundation for sentiment analysis. By combining structured data (e.g., numbers and statistics) with unstructured data (e.g., social media posts), companies can generate more accurate and contextualized reports.
Artificial Intelligence and Machine Learning
Machine learning algorithms allow sentiment analysis to evolve continuously, improving its accuracy over time. They also help identify nuances, such as sarcasm and irony, that previously went unnoticed.
Blockchain for Transparency
In the future, data transparency will be a competitive advantage. Blockchain can ensure that the analyzed information is reliable and free from manipulation.
The Impact of Sentiment Analysis on Corporate Communication
Beyond its use in economic reports, sentiment analysis is also revolutionizing how companies communicate with the public. By understanding consumer perceptions in real time, organizations can adapt their communication strategies to mitigate reputation crises or seize engagement opportunities.
Key Sentiment Analysis Tools Available in the Market
Currently, a wide variety of tools make sentiment analysis accessible and efficient, catering to different needs in the economic and corporate spheres.
- Google Cloud Natural Language AI:
A powerful tool that analyzes the tone of large-scale texts, widely used by technology companies and startups. - IBM Watson Tone Analyzer:
Focused on understanding the sentiment behind emails, messages, and posts, helping brands improve communication with their customers. - Hootsuite Insights:
Specialized in social media, it provides detailed sentiment analyses about public perception of campaigns and events.
These tools make sentiment analysis more accessible for organizations of all sizes.
Sentiment and Economy: A Powerful Duo
Sentiment analysis is not just an advanced technology; it bridges the gap between what people feel and the decisions that shape the economic world. When combined with predictive analytics, this tool goes beyond interpreting the present, projecting future scenarios based on collective emotions.
As we move towards an increasingly data-driven economy, understanding the “market mood” will be essential for making assertive decisions amidst uncertainty. After all, at the heart of every economic decision lies a touch of emotion—and now, we have the right tools to decode it and turn it into action.
References
IBM. Sentiment Analysis: Definition and Applications.
Análise Macro. Market Sentiment Analysis with AI Using Python.
UFF. Sentiment Analysis for Financial Market Trends.
ARXIV. Sentiment Dynamics in Financial Markets.
ARXIV. Sentiment Analysis with Neural Networks.
ARXIV. Advanced Sentiment Analysis for Predictive Models.
UNITE AI. Is Sentiment Analysis Effective in Predicting Trends in Financial Markets?.